Facefilter: FaceFilter для Windows — Скачайте бесплатно с Uptodown
FaceFilter PRO 3.05,фотографии
Компания Reallusion выпустила новую версию своей программы для ретуши фотографий FaceFilter3. На основе шаблонов система позволяет пользователям быстро и легко применять естественно выглядящие варианты макияжа для каждого конкретного лица с учетом его особенностей, а затем настроить и сохранить их для использования в будущем. Возможность выборочного морфинга и коррекция индивидуальных черт лица позволяет внести небольшие изменения в выражение лице.Reallusion FaceFilter 3 — передовая программа для фото-ретуширования, основанная на реальной теории макияжа. С помощью одного клика, вы можете применить шаблоны косметики, чтобы добиться идеального вида. Сделать естественный безупречный цвет лица, сглаживания кожи и инструменты удаления изъянов и улучшение, применяя пользовательский контуры и выделения слоев, чтобы подчеркнуть привлекательные черты лица. Универсальная система макияж глаз дает реалистичные текстуры волос ресниц и бровей, и объемные мышцы на основе морфинга лица, система изменения функции и повышения выразительности.
Основные особенности программы:
- Легкая и полная среда редактирования;
- Редактирование без потерь качества;
- Четыре различных режима просмотра, чтобы увидеть разницу;
- Точные результаты в деталях лица;
- Более 400 визуальных шаблонов изменения;
- Улучшенный режим сканирования;
- Экспорт Изображение с высоким разрешением.
- Регулировка тона цвета кожи;
- Работа с жирной кожей;
- Гладкая текстура кожи;
- Глаз;
- Шеи и плеч.
- Выделение Контуров для Sculpted;
- сияние красивого румянца;
- Дизайн и Полный макияж глаз;
- Нанесите помады и отбеливание зубов.
- Изменение формы и выражения:
- В один клик Применение шаблона;
- Расширенные настройки.
- Умная цветокоррекция;
- Применение Эффектов с объектами Фильтра;
- Создание великолепные эффекты DSLR;
- Улучшение фотографии.
Image output: (jpg, tif and raw)
Export: JPG или TIF
Что нового:
Version 3.05:- Release notes were unavailable when this listing was updated.
Снимки экрана:
Похожие новости
- Movavi Screen Recorder Studio 10.1.0
- Stellar Drive Clone 3.5.0.5
- Red Giant Magic Bullet Suite 12.1.6
- Radium 3.0.5 — интернет-радио плеер для Mac OS
- TurnTable 3.2.2
- PDF ePub DRM Removal — 1.6.0 (MacOSX)
- Live Home 3D Pro 3.5.3
- SimpleKeys 2.5.5 — cоздать сочетания клавиш для ваших общих задач
- Inpaint 5.
6 для Mac OS
- NeoOffice 2015.12
- iFoto Denoise 2.5
- Microsoft Office Standard 2019 v16.22.0
- Aurora 3D Text & Logo Maker 1.42.16
- TranslationPractice 1.1
- TunesKit Audio Converter 3.0.1.40
- Бред Хасси. Курс верстки PSD в HTML5/CSS3 (2014)
- One Chat — All In One Messenger 4.8
- Pillars of Eternity II: Deadfire (2018)
- Aegis 1.50
- Business Card Shop 7.0.2
Теги
- фотографии
[PDF] FaceFilter: Face Identification with Deep Learning and Filter Algorithm
- DOI:10.1155/2020/7846264
- Corpus ID: 221698713
@article{Alghaili2020FaceFilterFI, title={FaceFilter: Face Identification with Deep Learning and Filter Algorithm}, author={Mohammed Alghaili and Zhiyong Li and Hamdi A.R. Ali}, journal={Sci. Program.}, year={2020}, volume={2020}, pages={7846264:1-7846264:9} }
- Mohammed Alghaili, Zhiyong Li, H. A. Ali
- Published 1 August 2020
- Computer Science
- Sci. Program.
Although significant advances have been made recently in the field of face recognition, these have some limitations, especially when faces are in different poses or have different levels of illumination, or when the face is blurred. In this study, we present a system that can directly identify an individual under all conditions by extracting the most important features and using them to identify a person. Our method uses a deep convolutional network that is trained to extract the most important…
An Efficient Face Detection and Recognition System Using RVJA and SCNN
- P. Janarthanan, V. Murugesh, N. Sivakumar, S. Manoharan
- 2022
Computer Science
Mathematical Problems in Engineering
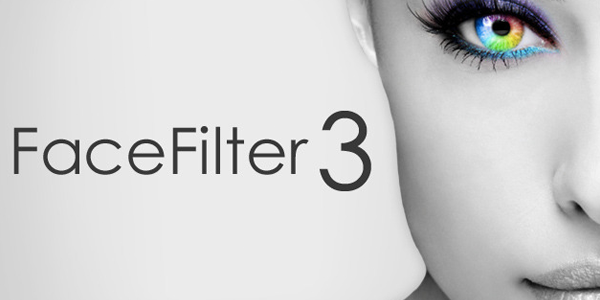
Efficient Face Recognition System for Operating in Unconstrained Environments
- Alejandra Sarahi Sanchez-Moreno, J. Olivares-Mercado, Aldo Hernandez-Suarez, K. Toscano-Medina, G. Sánchez-Pérez, Gibran Benitez-Garcia Computer Science
- 2021
J. Imaging
Face Recognition System Design and Implementation using Neural Networks
- Jamil Abedalrahim Jamil Alsyayadeh, I. -, A. Aziz, Chang Kai Xin, A. Hossain, S. Herawan
- 2022
Computer Science
International Journal of Advanced Computer Science and Applications

Analysis of Random Local Descriptors in Face Recognition
- Airam Curtidor, Tetyana Baydyk, E. Kussul
- 2021
Computer Science
Electronics
JULive3D: a live image acquisition protocol for real-time 3D face recognition
- P. Bagchi, D. Bhattacharjee
- 2023
Computer Science
Multimedia Tools and Applications
Image Sketch Based Criminal Face Recognition Using Content Based Image Retrieval
- Adimas Adimas, S.
- 2021

Computer Science
Scientific Journal of Informatics
Face Antispoofing Method Using Color Texture Segmentation on FPGA
- Youngjun Moon, Intae Ryoo, Seokhoon Kim
- 2021
Computer Science
Secur. Commun. Networks
The structure of the local detector of the reprint model of the object in the image
- A. A. Kulikov
- 2021
Computer Science
Russian Technological Journal

A Heterogeneous Face Recognition Approach for Matching Composite Sketch with Age Variation Digital Images
- Chethana H.T., Trisiladevi C. Nagavi
- 2021
Computer Science
2021 Sixth International Conference on Wireless Communications, Signal Processing and Networking (WiSPNET)
Применение биометрических систем в технологиях идентификации лиц
- А. А. Куликов
- 2021
Computer Science
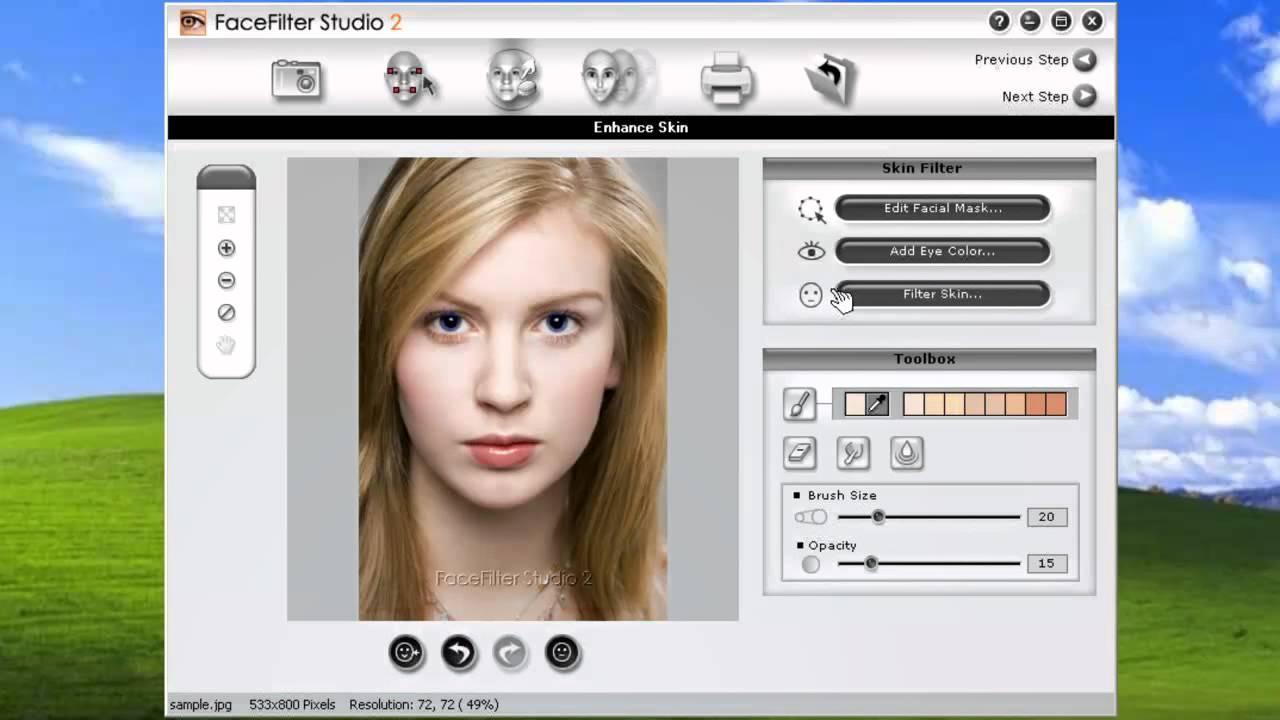
Deep Learning Identity-Preserving Face Space
- Zhenyao Zhu, Ping Luo, Xiaogang Wang, Xiaoou Tang
- 2013
Computer Science
2013 IEEE International Conference on Computer Vision
Deep Learning Face Representation by Joint Identification-Verification
- Yi Sun, Yuheng Chen, Xiaogang Wang, Xiaoou Tang
- 2014
Computer Science
NIPS
FaceNet: A unified embedding for face recognition and clustering
- Florian Schroff, Dmitry Kalenichenko, James Philbin
- 2015
Computer Science
2015 IEEE Conference on Computer Vision and Pattern Recognition (CVPR)

DeepFace: Closing the Gap to Human-Level Performance in Face Verification
- Yaniv Taigman, Ming Yang, M. Ranzato, Lior Wolf
- 2014
Computer Science
2014 IEEE Conference on Computer Vision and Pattern Recognition
Learning Face Representation from Scratch
- Dong Yi, Zhen Lei, Shengcai Liao, S. Li
- 2014
Computer Science
ArXiv

Web-scale training for face identification
- Yaniv Taigman, Ming Yang, M. Ranzato, Lior Wolf
- 2015
Computer Science
2015 IEEE Conference on Computer Vision and Pattern Recognition (CVPR)
Deep Learning Face Representation from Predicting 10,000 Classes
- Yi Sun, Xiaogang Wang, Xiaoou Tang
- 2014
Computer Science
2014 IEEE Conference on Computer Vision and Pattern Recognition
Deep Face Recognition
- O. Parkhi, A. Vedaldi, Andrew Zisserman
- 2015
Computer Science
BMVC

Deep Learning Face Attributes in the Wild
- Ziwei Liu, Ping Luo, Xiaogang Wang, Xiaoou Tang
- 2015
Computer Science
2015 IEEE International Conference on Computer Vision (ICCV)
Face recognition in unconstrained videos with matched background similarity
- Lior Wolf, Tal Hassner, I. Maoz
- 2011
Computer Science
CVPR 2011
|
|
|
FaceFilter: аудиовизуальное разделение речи с использованием неподвижных изображений
- Чанг, Су-Ван ;
- Чхве, Соён ;
- Сон Чунг, Джун ;
- Кан, Хонг-Гу
Аннотация
Цель этой статьи состоит в том, чтобы отделить речь целевого говорящего от смеси двух говорящих, используя сеть глубокого аудиовизуального разделения речи. В отличие от предыдущих работ, в которых в качестве вспомогательного условного признака использовалось движение губ на видеоклипах или предварительно зарегистрированная информация о говорящем, мы используем изображение одного лица целевого говорящего. В этой задаче условный признак получается из внешнего вида лица в кросс-модальной биометрической задаче, где звуковые и визуальные представления идентичности совместно используются в скрытом пространстве. Идентификация, полученная по изображениям лиц, заставляет сеть изолировать совпадающих говорящих и извлекать голоса из смешанной речи. Это решает проблему перестановки, вызванную перестановкой выходных каналов, часто возникающую в задачах разделения речи. Предлагаемый метод гораздо более практичен, чем разделение речи на основе видео, поскольку изображения профилей пользователей легко доступны на многих платформах. Кроме того, в отличие от методов разделения с учетом говорящих, он применим при разделении с невидимыми говорящими, которые никогда ранее не регистрировались. Мы показываем сильные качественные и количественные результаты на сложных примерах из реальной жизни.
- Публикация:
Электронные распечатки arXiv
- Дата публикации:
- Май 2020
- DOI:
- 10.